Machine Learning and AI in Neuroimaging Analysis
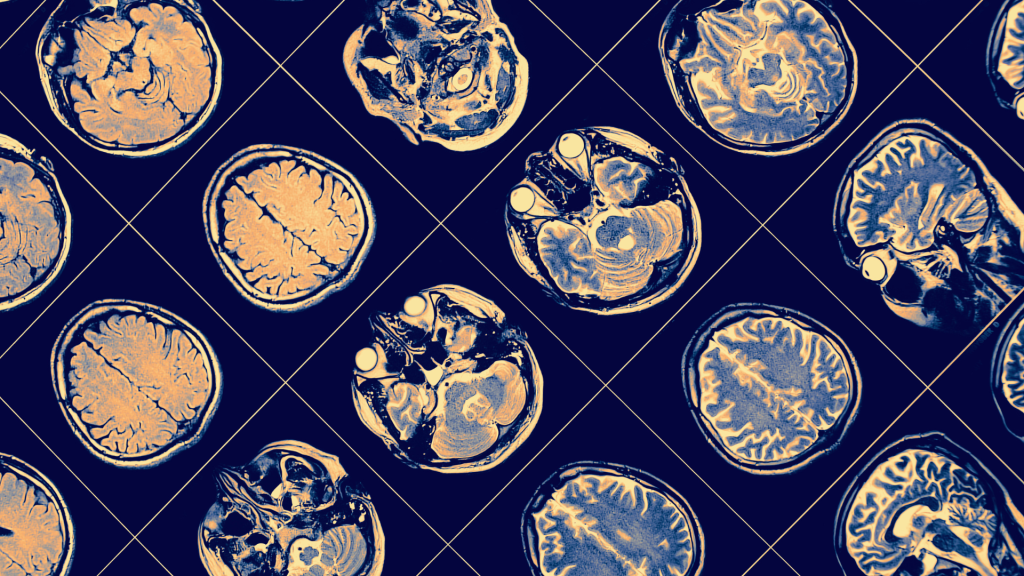
Machine Learning and AI in Neuroimaging Analysis: Automated Interpretation and Predictive Analytics
Objective:
To explore the role of machine learning (ML) and artificial intelligence (AI) in advancing neuroimaging analysis, focusing on automated interpretation and predictive analytics.
Introduction:
Machine learning and AI have made significant contributions to the field of neuroimaging by automating data processing and enhancing the interpretive capabilities of neuroimaging techniques. These technologies are increasingly being used to analyze large-scale neuroimaging datasets, extract meaningful patterns, and make predictions about brain health, disease progression, and treatment outcomes.
Key Concepts in ML and AI for Neuroimaging:
- Automated Image Analysis:
- ML algorithms, such as deep learning models, are used to automatically segment brain images, classify brain structures, and detect abnormalities. These algorithms can identify patterns in the data that might be missed by human interpreters.
2. Predictive Analytics:
- AI is used to predict outcomes based on neuroimaging data. For example, AI models can predict the onset of neurodegenerative diseases or assess how a brain injury will affect recovery based on imaging data.
3. Neuroimaging Biomarkers:
- AI and ML techniques can identify biomarkers in neuroimaging data that are associated with specific conditions, such as Alzheimer’s or schizophrenia, facilitating early diagnosis and personalized treatment strategies.
Applications of Machine Learning and AI in Neuroimaging:
Early Diagnosis of Neurodegenerative Diseases:
- Machine learning algorithms can analyze patterns in brain images to detect early signs of conditions like Alzheimer’s and Parkinson’s disease, enabling earlier interventions and personalized treatment plans.
Personalized Medicine:
- AI can analyze individual patient data and predict how a patient’s brain will respond to specific treatments, facilitating a more personalized approach to care.
Monitoring Disease Progression:
- AI models can track changes in brain structures over time, providing valuable insights into how diseases such as multiple sclerosis or traumatic brain injury (TBI) evolve.
Real-World Example:
- Predicting Alzheimer’s Disease:
- Researchers have developed AI models that analyze MRI scans to predict the likelihood of a patient developing Alzheimer’s disease, years before clinical symptoms appear.
Case Study:
Stroke Recovery:
- AI models have been used to predict recovery outcomes in stroke patients by analyzing post-stroke brain scans. These predictions help in personalizing rehabilitation plans and improving patient outcomes.